MInDS@Mines Undergraduate Researchers Present at the 2019 Undergraduate Research Symposium
Sun Apr 28, 2019
Zoe Baker and Madeline McKune, two undergraduate student researchers on the MInDS team, presented their work on effective visualization of machine learning results at the annual Undergraduate Research Symposium at CoorsTek.
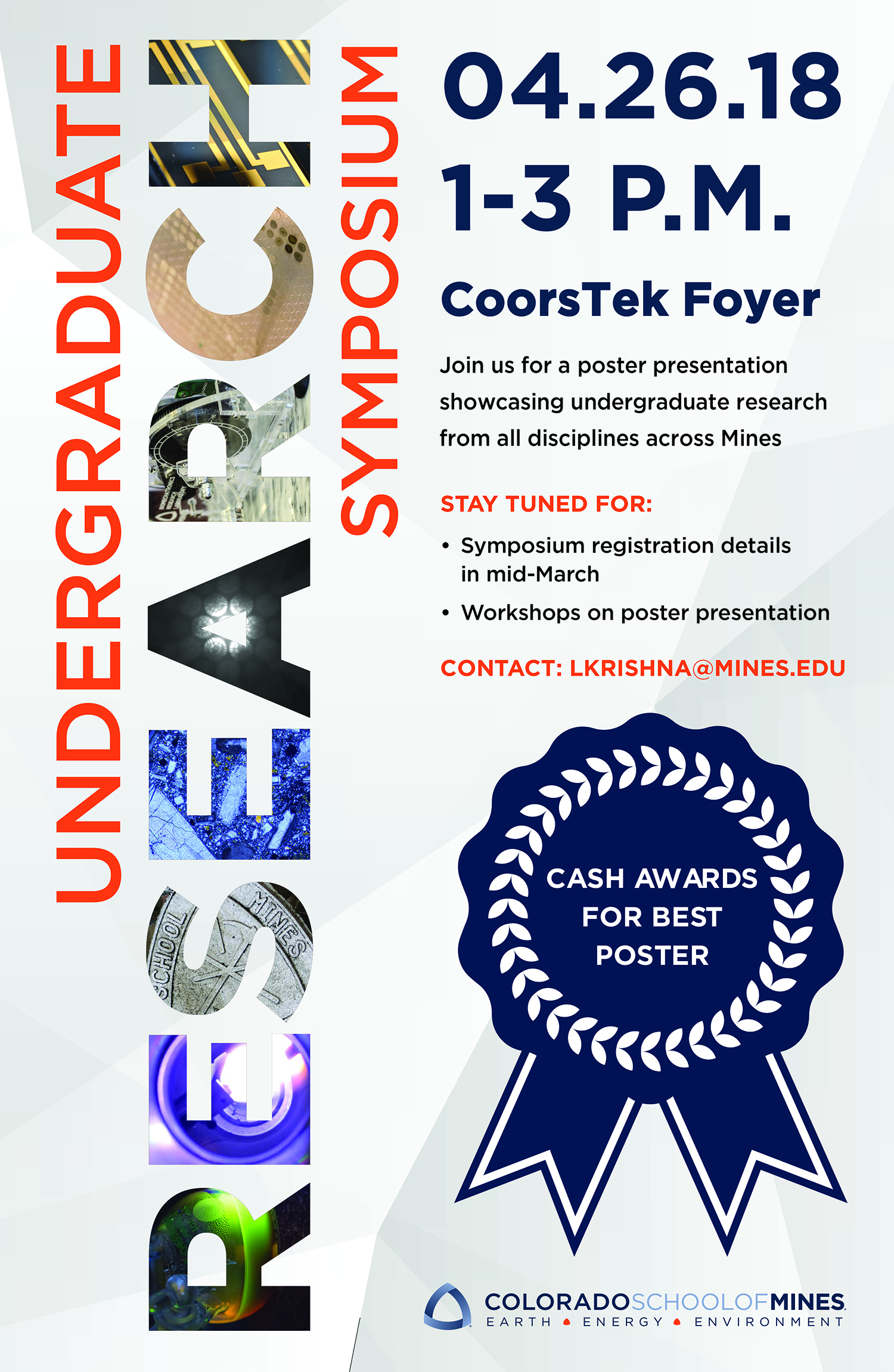
The annual Undergraduate Research Symposium provides a forum for Mines students to present their research to the entire community at the Colorado School of Mines.
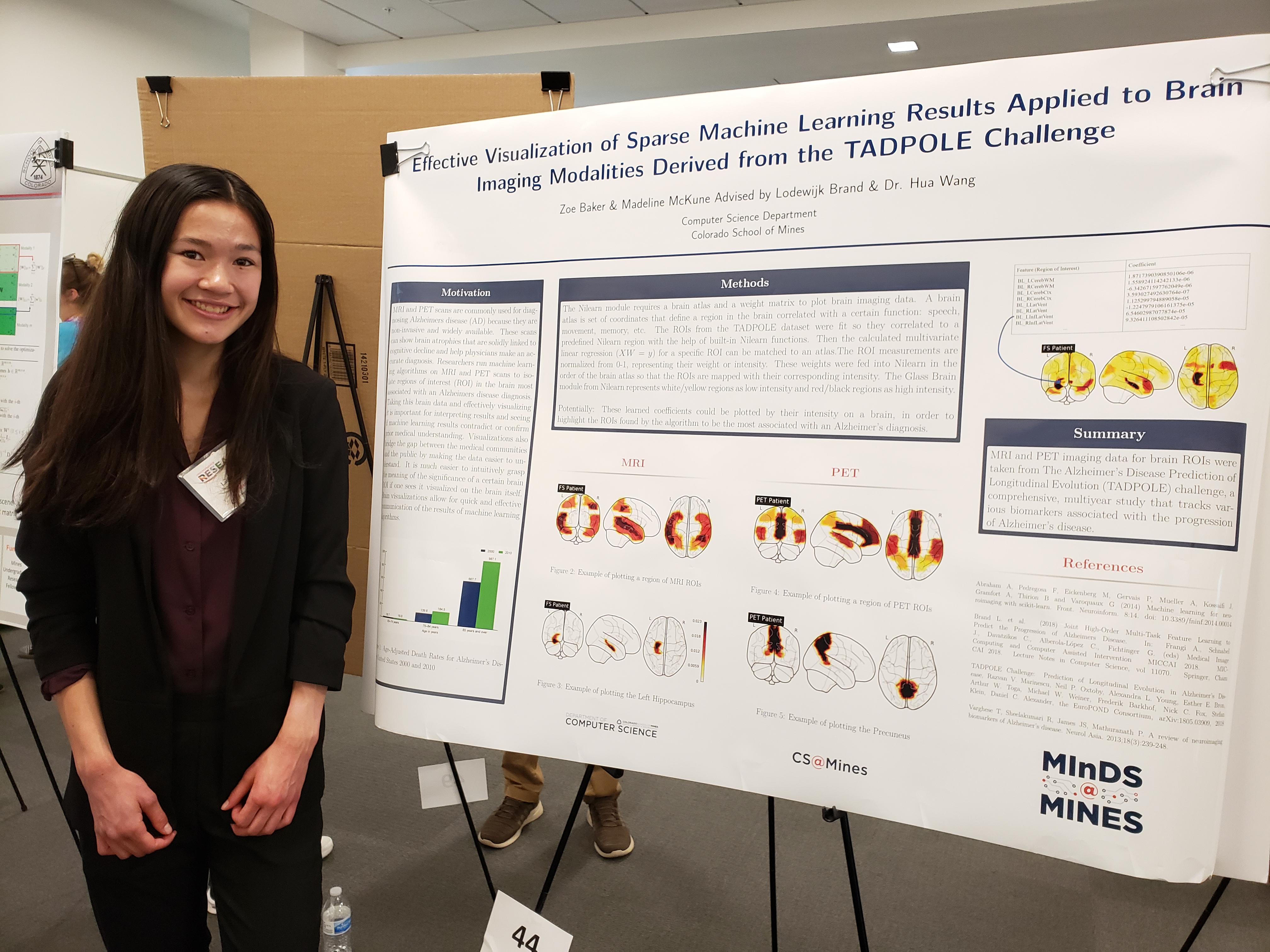
Zoe's poster titled Effective Visualization of Sparse Machine Learning Results Applied to Brain Imaging Modalities Derived from the TADPOLE Challenge.
Abstract: MRI and PET scans are commonly used for diagnosing Alzheimer’s disease (AD) because they are non-invasive and widely available. These scans can show brain atrophies that are solidly linked to cognitive decline and help physicians make an accurate diagnosis. Researchers run machine learning algorithms on MRI and PET scans to isolate regions of interest (ROI) in the brain most associated with an Alzheimer’s disease diagnosis. Taking this brain data and effectively visualizing it is important for interpreting results and seeing if machine learning results contradict or confirm prior medical understanding. Visualizations also bridge the gap between the medical communities and the public by making the data easier to understand. For example, hearing that a region of the brain is affected vs seeing that region, or related regions of the brain, is lit up. Brain visualizations allow for quick and effective communication of the results of machine learning algorithms.
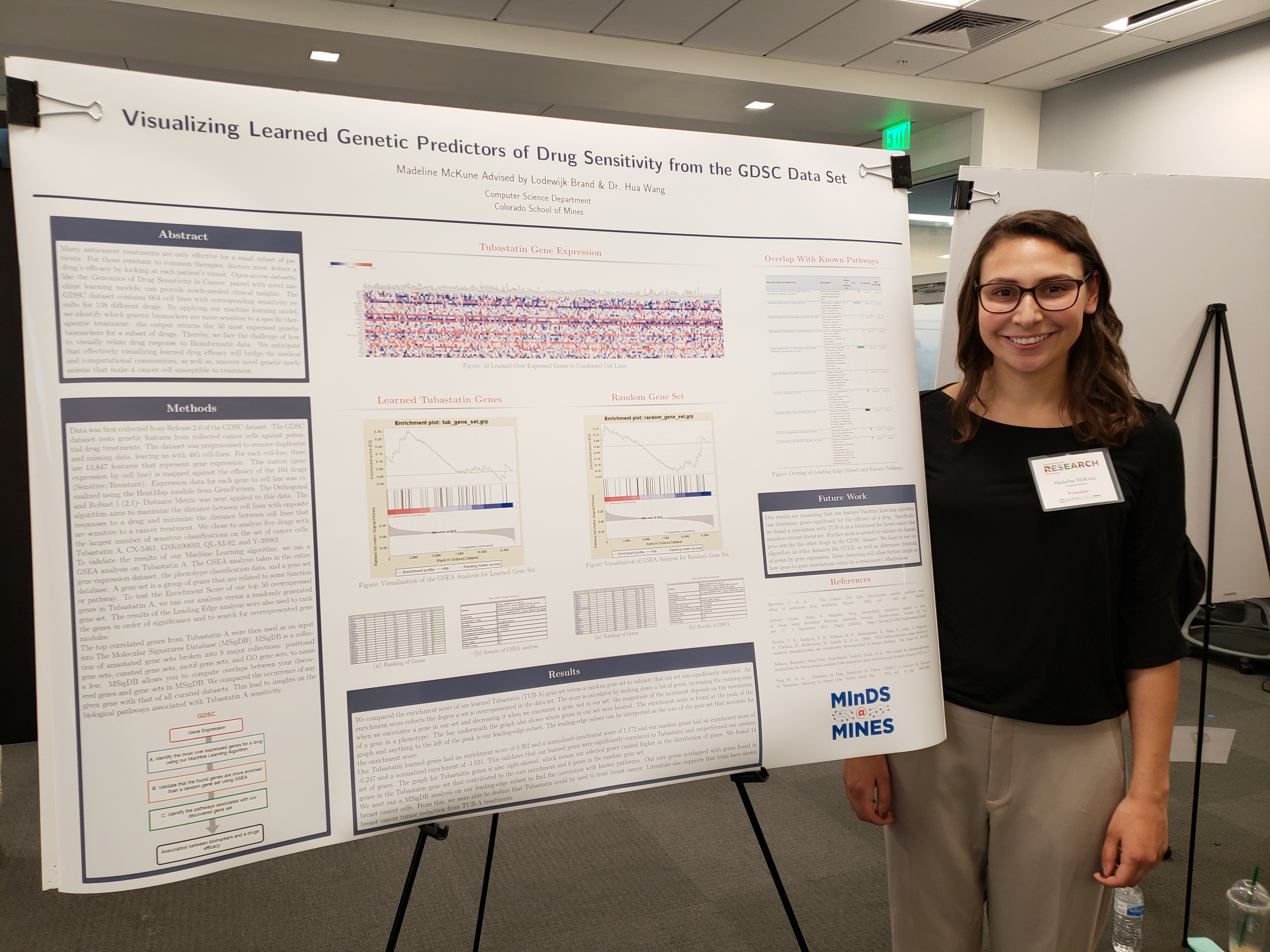
Madeline's poster titled Visualizing Learned Genetic Predictors of Drug Sensitivity from the GDSC Data Set.
Abstract: Many anticancer treatments are only effective for a small subset of patients. For those resistant to common therapies, doctors must deduce a drug’s efficacy by looking at each patient’s tumor. Open-access datasets, like the Genomics of Drug Sensitivity in Cancer, paired with novel machine learning models, can provide much-needed clinical insights. The GDSC dataset contains 664 cell lines with corresponding sensitivity results for 138 different drugs. By applying our machine learning model, we identify which genetic biomarkers are more sensitive to a specific therapeutic treatment: the output returns the 50 most expressed genetic biomarkers for a subset of drugs. Thereby, we face the challenge of how to visually relate drug response to bioinformatic data. We anticipate that effectively visualizing learned drug efficacy will bridge the medical and computational communities, as well as, uncover novel genetic mechanisms that make a cancer cell susceptible to treatment.