Additive manufacturing (AM) and data-driven design are becoming increasingly common in a wide range of industries. Unlike plastics 3D printing, which can be done by hobbyists in their homes, AM of metals requires a high-level of skill, expertise, and specialized know-how to adjust and tune the manufacturing process for a specific part geometry, material, or desired functional outcome. As such, building a new part or changing to a new material with AM processing is currently time consuming, tedious, and expensive (on the order of 5 years and $5M for a single “A-Basis Allowable” part). Correspondingly, the economic uncertainty of launching innovative products or entering new, untested markets often renders AM cost-prohibitive. As AM has gained popularity in the last decade, this has been the most critical obstacle inhibiting its mass adoption as a production technique rather than as a rapid prototyping method.
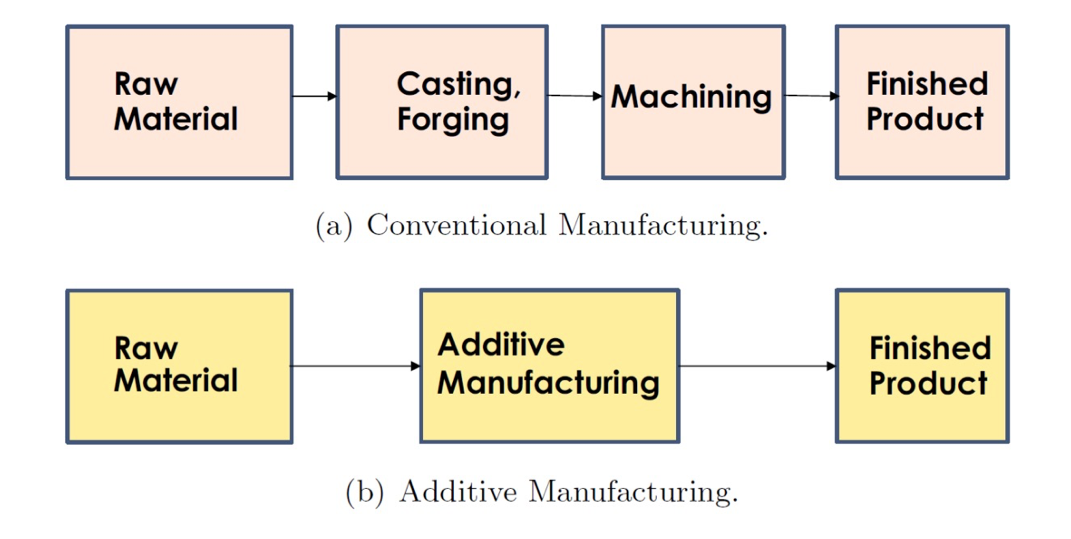
Figure 1: Comparison of manufacturing methods.
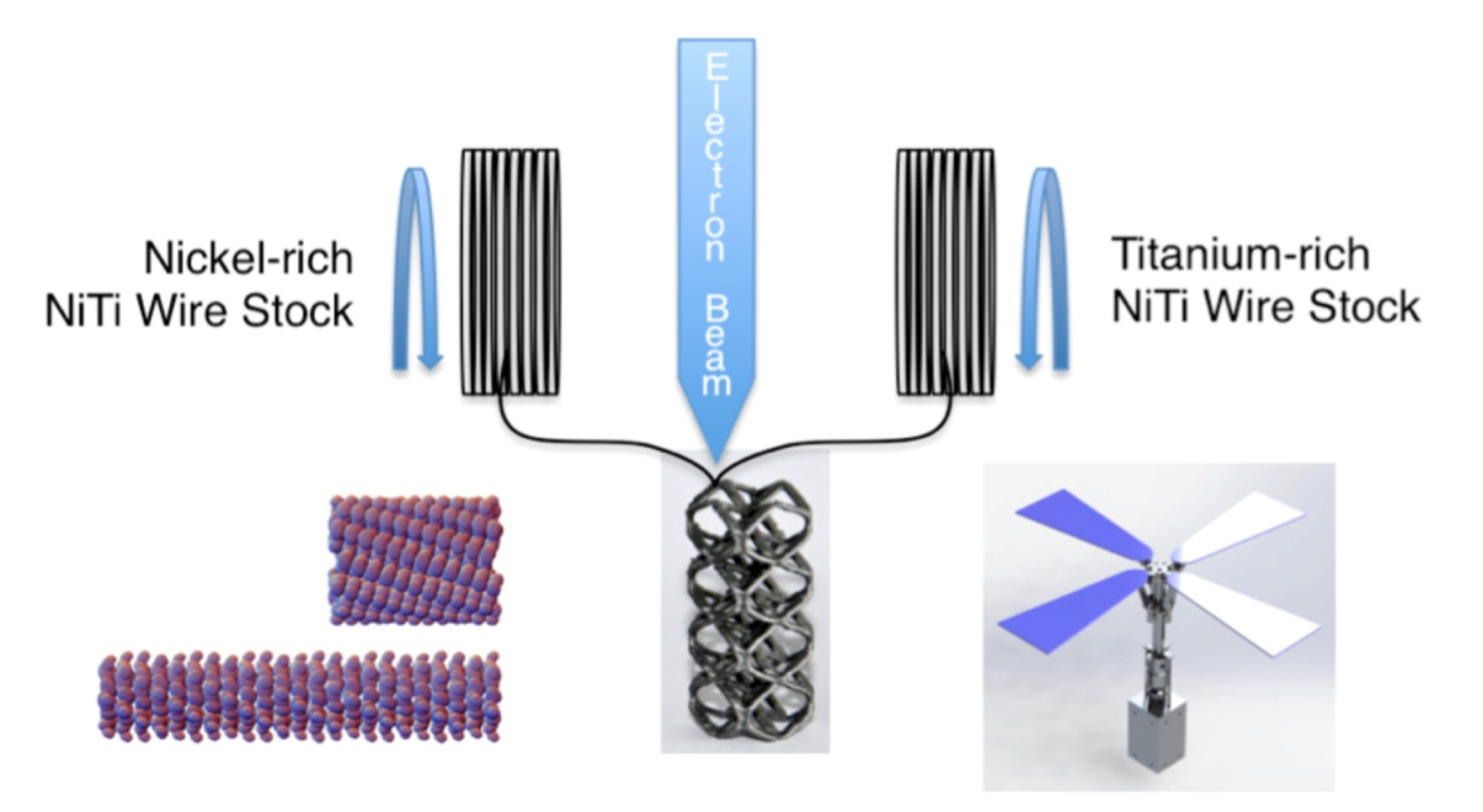
Figure 2: A fabrication process through additive manufacturing.
The objective of this project is to address the above critical problem by enabling manufacturers to efficiently employ AM processes to expand their product offerings and to provide resilient employment and value to society. Multiple machine learning approaches for process control will be developed. The most successful algorithms will then be integrated to create the final process model. Specifically, we will investigate how to predict the process settings that will result in desired materials properties and quantify model uncertainties.
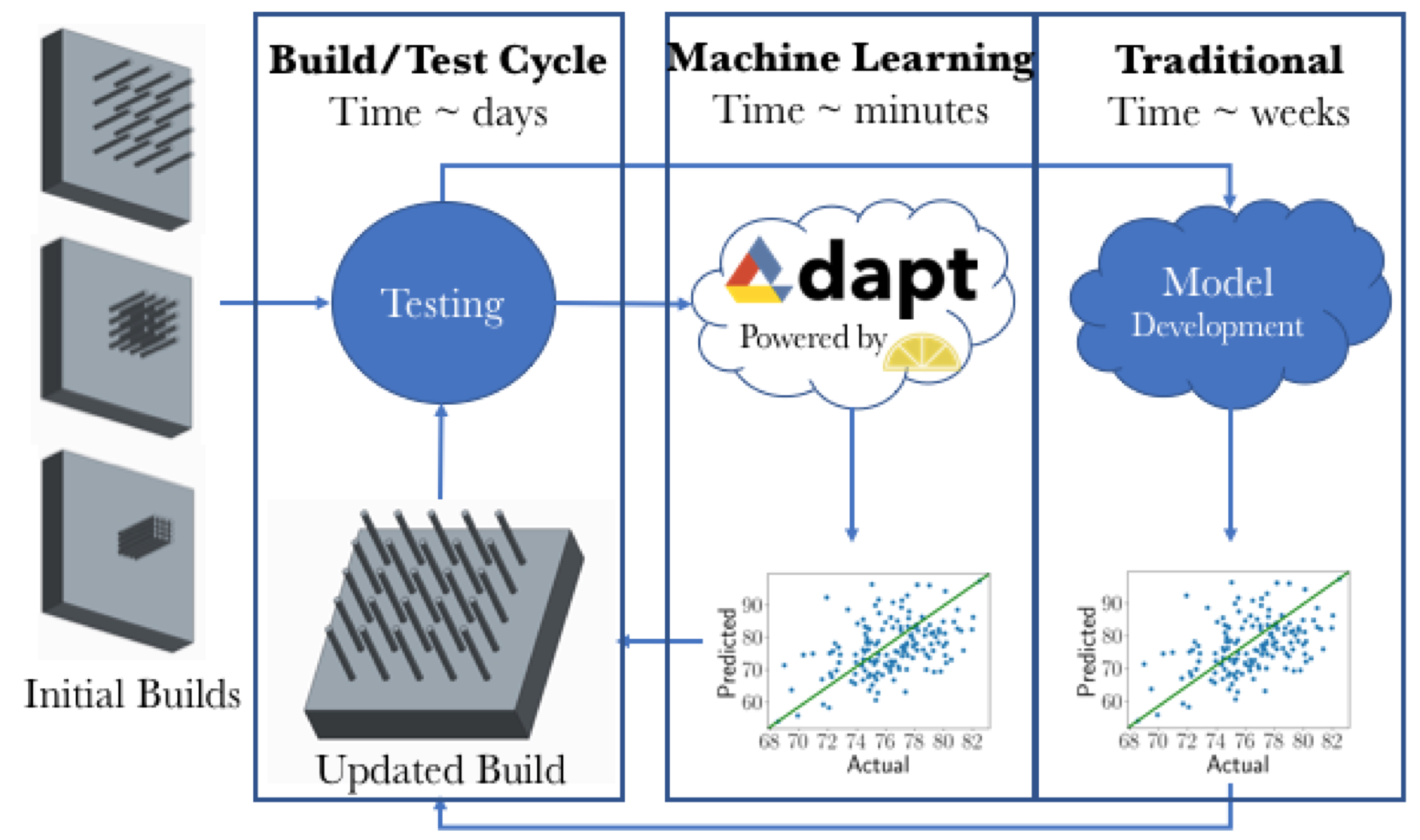